What is Blackbox AI, and how does it work?
- Hannah Matthew Adgale
- Mar 27
- 3 min read
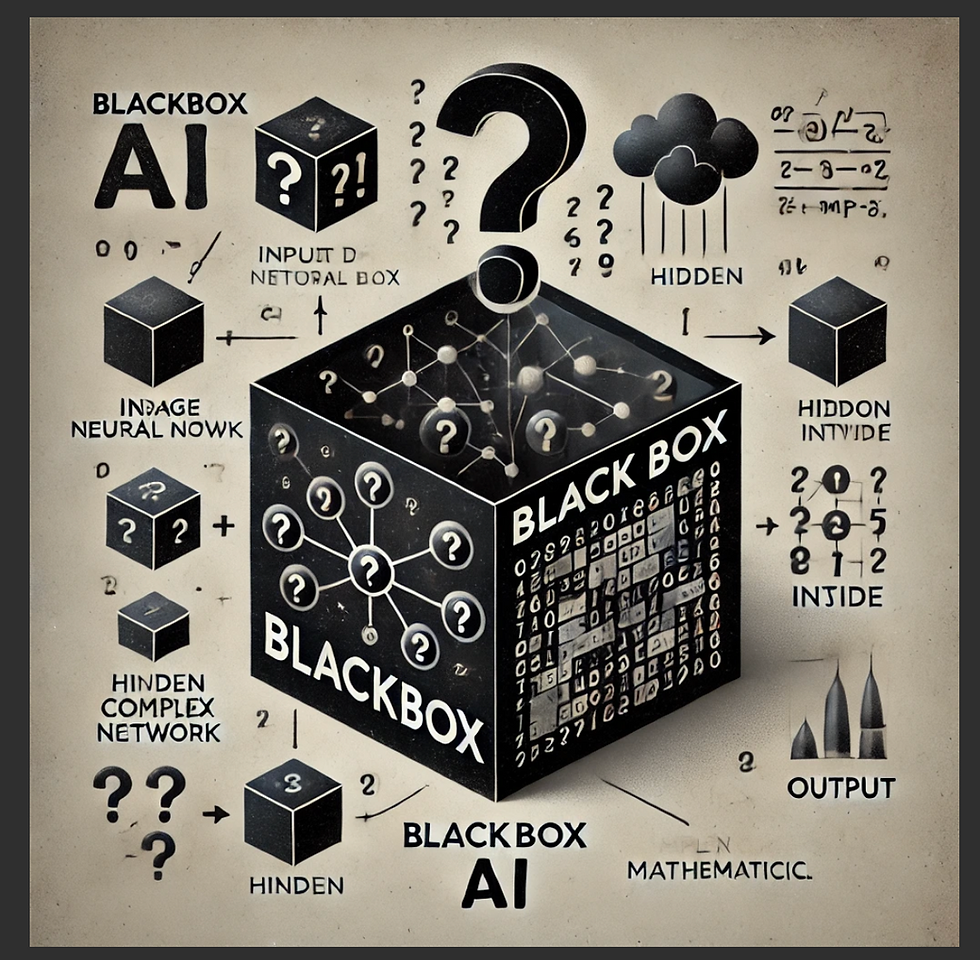
Blackbox AI is a type of artificial intelligence where humans do not easily understand the decision-making process. It takes input (like images, text, or numbers) and produces an output (such as predictions or classifications), but how it arrives at the result is unclear.
What is Blackbox AI?
Blackbox AI refers to artificial intelligence systems, particularly machine learning (ML) models, whose internal decision-making processes are not easily interpretable by humans. These AI models take inputs and produce outputs, but the reasoning behind their decisions remains unclear—hence the term "black box."
Blackbox AI is common in deep learning, where complex neural networks process vast amounts of data to make predictions. However, due to their intricate mathematical computations, it becomes difficult to explain why they make specific decisions.
How Blackbox AI Works
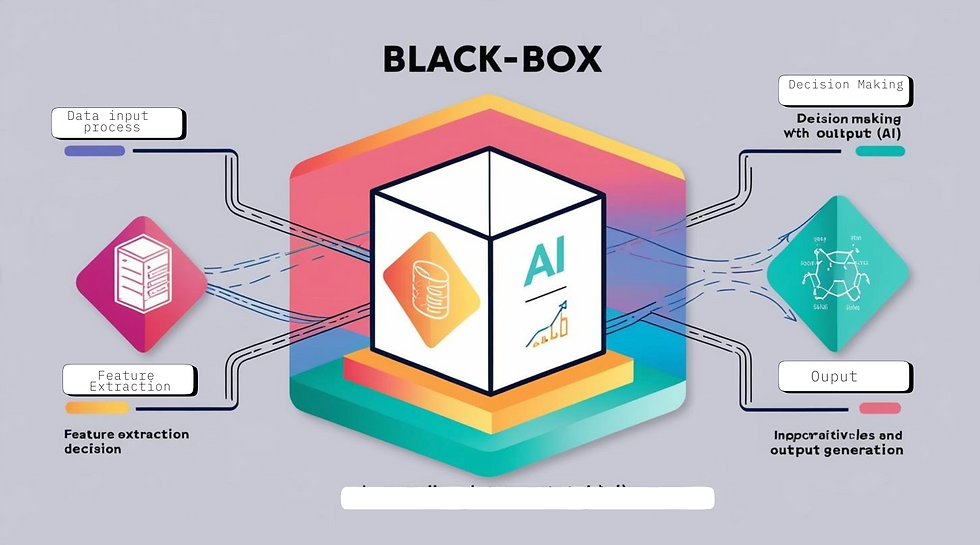
1. Data Input
The AI model receives raw input data, such as images, text, audio, or numerical values. This data is usually preprocessed to remove noise, standardize formats, and ensure better accuracy.
2. Feature Extraction
The AI system analyzes the input data and extracts relevant features—patterns or attributes that influence decision-making. In deep learning, hidden layers within neural networks automatically detect these features.
3. Decision-Making (Inference)
Using trained parameters (weights and biases), the AI model processes the extracted features through multiple layers of computation. These layers form a black box since humans cannot easily interpret how the model arrives at its final decision.
4. Output Generation
The AI system produces an output based on the learned patterns. For example:
A computer vision model classifies an image as a "cat" or "dog."
A language model translates a sentence from English to French.
A financial model predicts stock market trends.
5. Lack of Interpretability
Unlike traditional algorithms (such as decision trees or linear regression), blackbox AI does not provide a transparent reasoning path. If an AI predicts an individual is at high risk for loan default, it is difficult to determine the exact factors influencing this decision.
Examples of Blackbox AI
Deep Neural Networks (DNNs)
Used in image recognition, natural language processing (NLP), and autonomous driving.
Example: Google’s DeepMind models like AlphaGo.
Generative AI (e.g., GPT-4, DALL-E)
AI systems that generate text, images, or videos based on learned patterns.
Examples: ChatGPT, Midjourney.
Recommendation Systems
AI-powered content recommendations for Netflix, YouTube, and Spotify.
Example: AI suggests movies based on past viewing history, but the reasoning behind its recommendations remains unclear.
Medical Diagnosis AI
AI models detect diseases from medical scans but do not explain the reasoning behind a diagnosis.
Example: IBM Watson for Oncology.
Challenges of Blackbox AI
1. Lack of Transparency
Hard to interpret AI decisions.
Can lead to distrust in AI systems.
2. Bias and Ethical Issues
AI can learn biased patterns from training data.
May result in discrimination in hiring, loans, and legal judgments.
3. Accountability & Regulation
Difficult to assign responsibility for AI errors.
Governments push for explainable AI (XAI) to improve transparency.
Conclusion
Blackbox AI has revolutionized various industries by enabling powerful predictive capabilities, automation, and data-driven decision-making. However, its lack of interpretability raises concerns about trust, bias, and accountability. As AI continues to evolve, researchers and policymakers are working on Explainable AI (XAI) techniques to make these systems more transparent and understandable. Striking a balance between AI’s complexity and the need for interpretability is crucial to ensuring ethical and responsible AI deployment in the future.
Comments